Based on the development of the intelligent driving vehicles and the related key technologies, systematic interdisciplinary research has been carried out from the communications security, laser radar, machine vision, active safety, energy saving such aspects.
1.Perception
Research on the information fusion technology of the vehicle radar and machine vision and new technology of space 3D information construction in visual detection and measurement technology
2.Autonomous driving decision
Based on the framework of deep reinforcement learning, to construct the automatic driving strategy learning model and to improve the training efficiency using parallel computing.
3.Car network communication security
Research on the encryption and decryption, identity authentication and key distribution of vehicle network access, safe driving, billing management, traffic management and data communication.
4.Energy-saving driving
Through the path planning and speed adjustment to achieve energy-saving, combined with large traffic data, so as to achieve energy conservation from the city level.
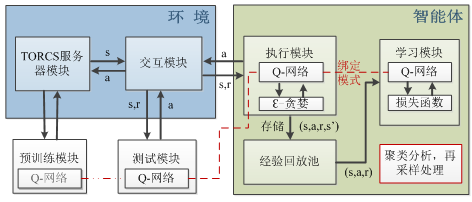
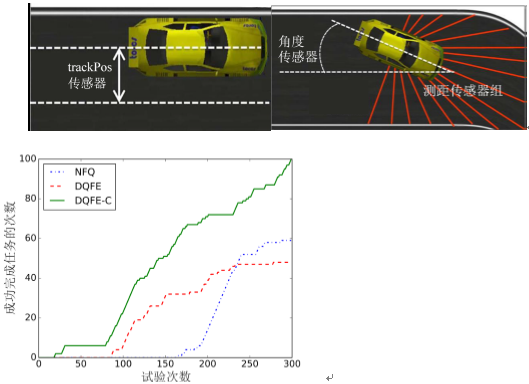
Selected Papers
1.Junta Wu, Huiyun Li*, Deep Ensemble Reinforcement Learning with Multiple Deep Deterministic Policy Gradient Algorithm, Mathematical Problems in Engineering, vol. 2020, Article ID 4275623, 12 pages, 2020 (IF= 1.179, SCI, JCR Q3)
2.夏伟,李慧云*,基于深度强化学习的自动驾驶策略学习方法,集成技术,第6卷 第3期,2017 年5 月
Selected Patents
1.发明专利,一种汽车的自动驾驶方法与装置,申请号:201710156331.8,申请日:2017.3.16
2.发明专利,一种用于车辆自动驾驶的决策网络模型的生成方法及装置,申请号:201710201086.8,申请日:2017.3.30