Based on the development of the intelligent driving vehicles and the related key technologies, systematic interdisciplinary research has been carried out from the communications security, laser radar, machine vision, active safety, energy saving such aspects.
1.Perception
Research on the information fusion technology of the vehicle radar and machine vision and new technology of space 3D information construction in visual detection and measurement technology
2.Autonomous driving decision
Based on the framework of deep reinforcement learning, to construct the automatic driving strategy learning model and to improve the training efficiency using parallel computing.
3.Car network communication security
Research on the encryption and decryption, identity authentication and key distribution of vehicle network access, safe driving, billing management, traffic management and data communication.
4.Energy-saving driving
Through the path planning and speed adjustment to achieve energy-saving, combined with large traffic data, so as to achieve energy conservation from the city level.
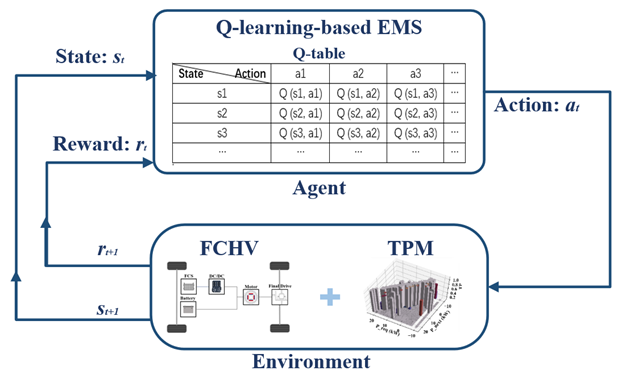
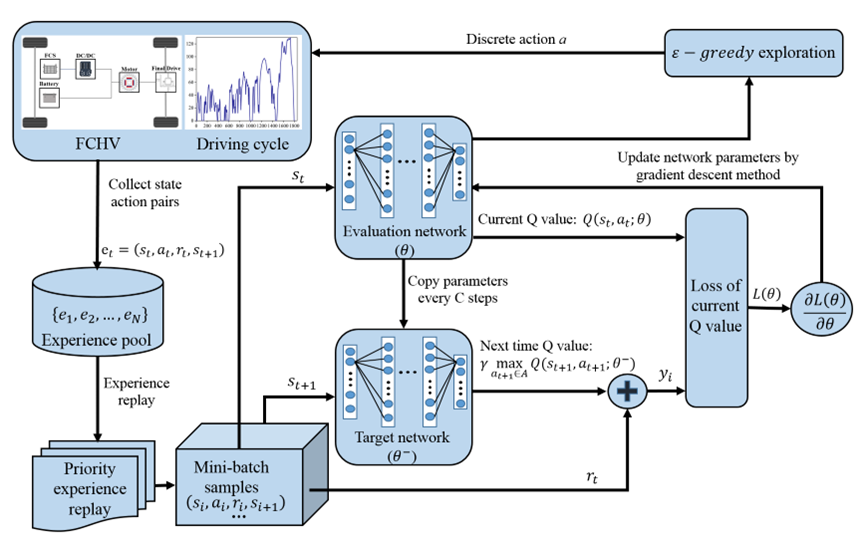
Selected Papers
[1] Chunhua Zheng*, Dongfang Zhang, Yao Xiao, Wei Li. Reinforcement learning-based energy management strategies of fuel cell hybrid vehicles with multi-objective control[J]. Journal of Power Sources, 2022, 543: 231841.
[2] Dezhou Xu, Yunduan Cui, Jiaye Ye, Suk Won Cha, Aimin Li, Chunhua Zheng*. A soft actor-critic-based energy management strategy for electric vehicles with hybrid energy storage systems[J]. Journal of Power Sources, 2022, 524: 231099.
[3] Chunhua Zheng, Wei Li, Weimin Li, Kun Xu, Lei Peng, Suk Won Cha*. A deep reinforcement learning-based energy management strategy for fuel cell hybrid buses[J]. International Journal of Precision Engineering and Manufacturing-Green Technology, 2022, 9(3): 885-897.
Selected Patents
(1)The invention relates to a method and equipment for verifying an automotive energy management strategy, China patent for invention, 2019/12/12
(2)The invention relates to a method and equipment for verifying an automotive energy management strategy, PCT patent, 2019/12/12